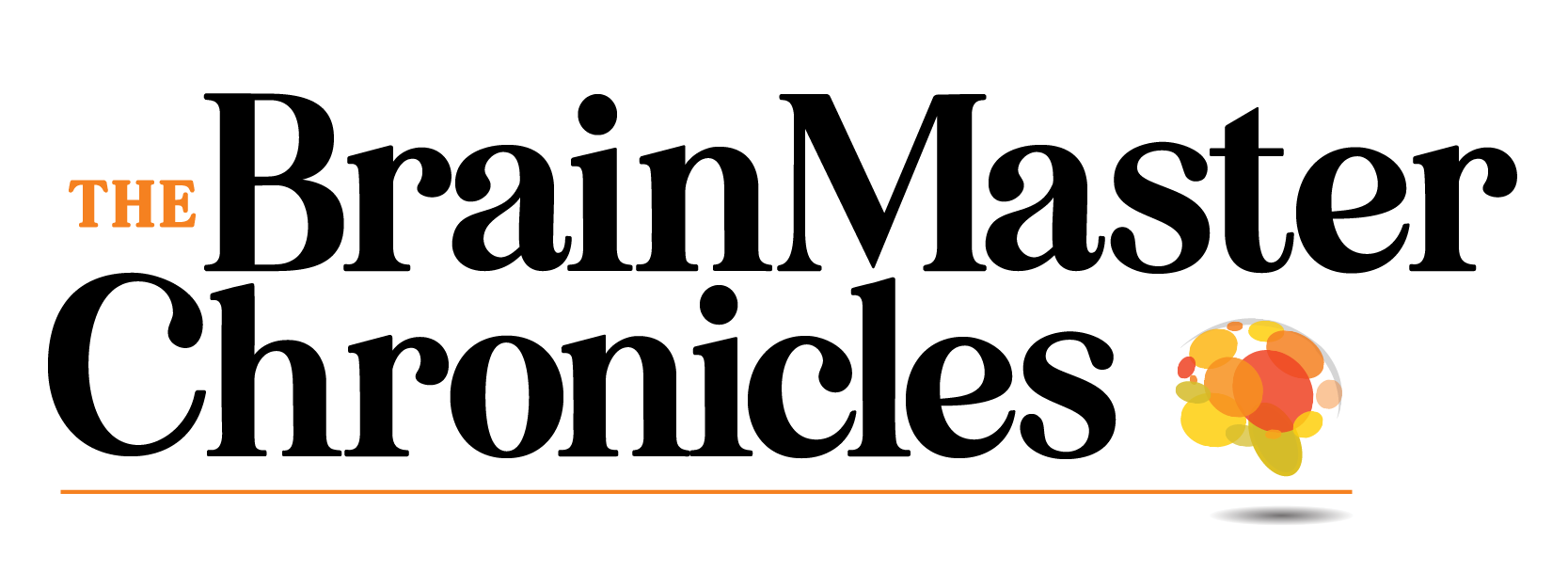
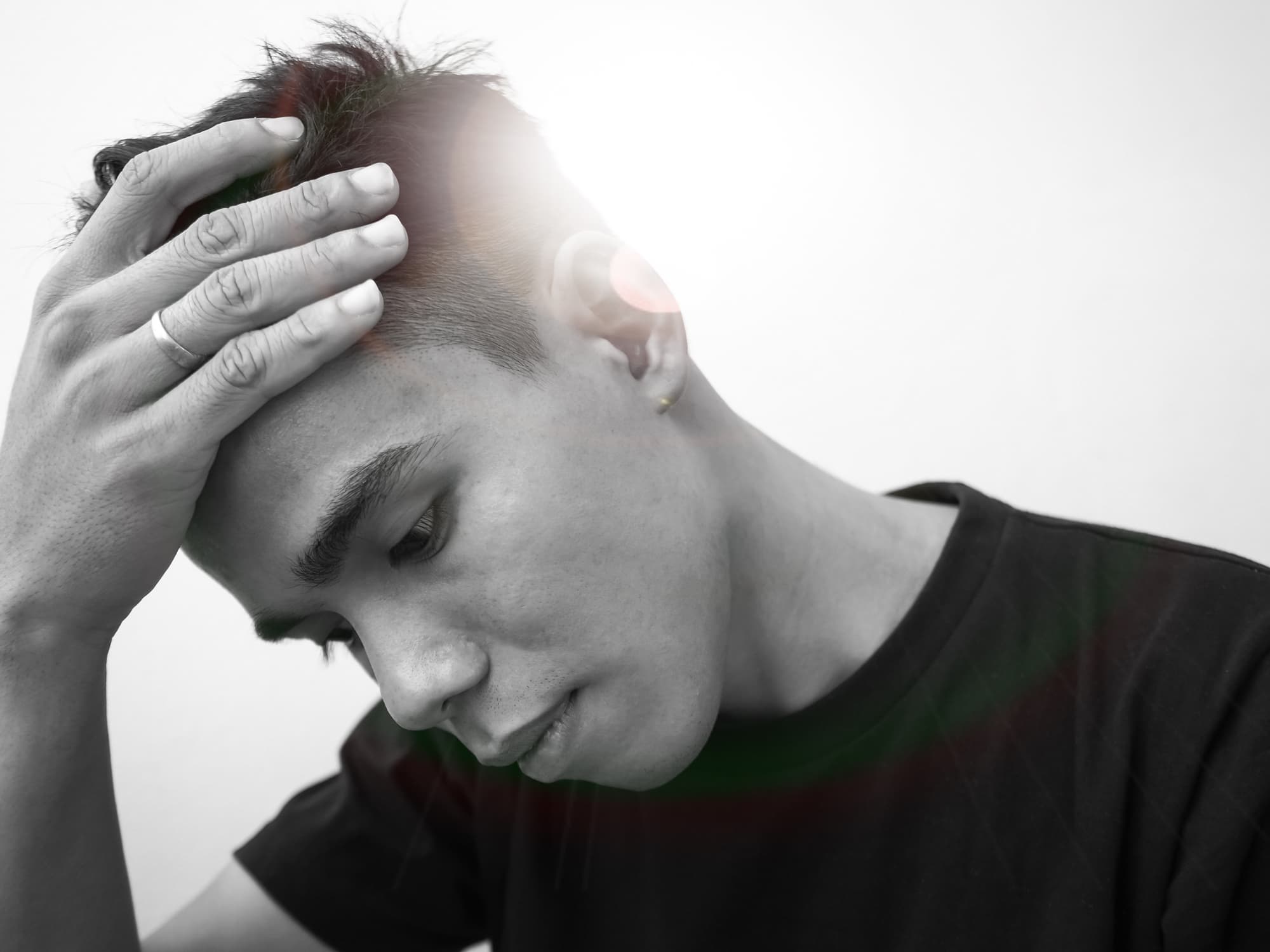
The Brain Panel
“Automation is good,
so long as you know exactly
where to put the machine.”
— Eliyahu Goldratt
The BrainMaster-designed Brain Panel is an example of a new method for fast screening EEGs. This new generation EEG analysis tool is now incorporated as part of the STS EEG Screening service, which also includes a signed report by a board certified M.D. neurologist.
We are teaching computers to examine an EEG and compare it with a large sample of other EEGs. It is not “normative” in that it is compared with clinical samples, not “normals.” The purpose of our system is to pre-screen EEGs that have not yet been inspected or artifacted, and determine how well they fit into a “typical” type of recording, as well as identifying phenotypes that may be evident. This report can provide a heads-up of what to look for when examining an EEG for purposes of clinical referral — or to prepare the recording for QEEG analysis. This is, therefore, a “pre-Q” or even a “pre-pre-Q.”
We have further enhanced our system to provide a report composed and formatted to replicate the physician’s quality review. Based on the quantitative findings derived from our metric analysis, we are able to determine how well this method actuallyproduces a useful report.
This process can be thought of as being similar to the familiar blood test panels, which provide an array of information related to blood health, compared to a clinical population. A clinician can review a blood panel with a client and explain the importance of each finding. The Brain Panel performs a similar role, but uses properties extracted mathematically from the EEG waves, in place of the chemical analysis used in a blood test. This report contains measurements relevant to the overall quality of the EEG recording, as well as the presence of deviations that may have possible clinical significance.
Based on this automated analysis of the brain wave data, a clinician can now share the Brain Panel with a client and help them understand what significant findings are present.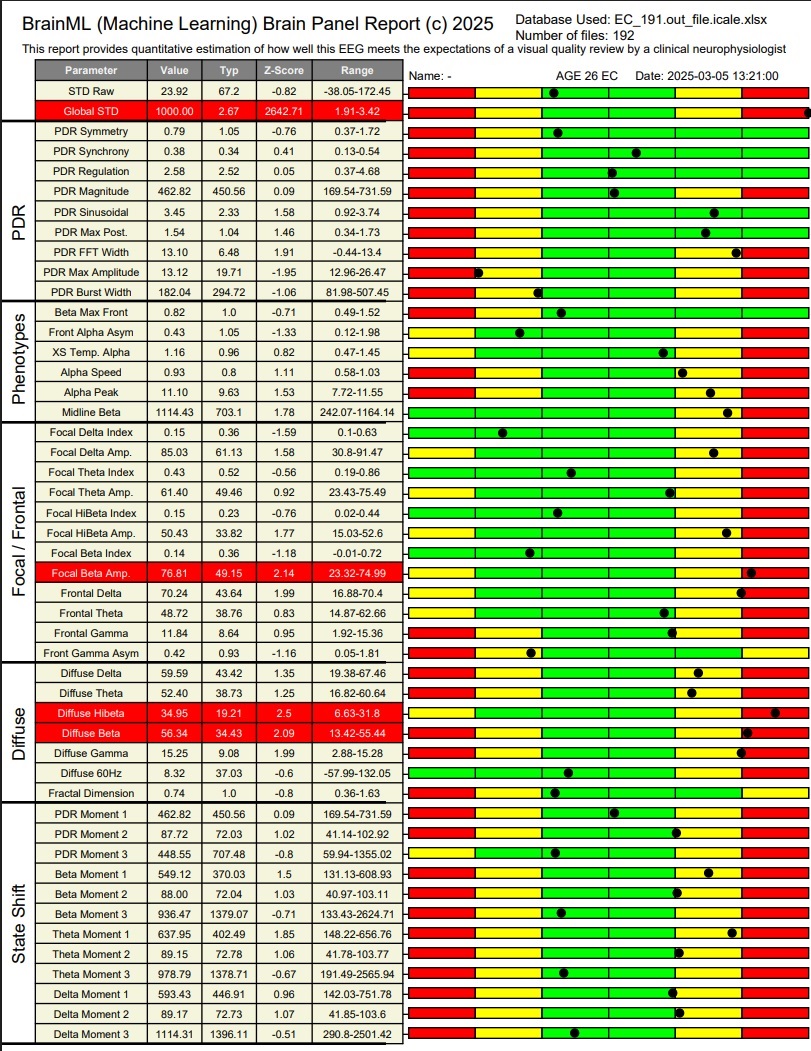
A summary of relevant detected deviations is also provided:
TECHNICAL BACKGROUND
In order to simulate what a doctor does when performing a visual inspection of an EEG, a computer is equipped with a program designed to use digital signal processing. We pick the rules and do not allow the machine to make decisions about which metrics or derived computations are used. We define a set of reasonably informed metrics motivated by the concepts of the posterior dominant rhythms, amplitude foci and magnitudes, time course of various metrics, and so on.
An ICA is applied to remove eye artifact. We analyze a series of 10-second epochs, providing a 0.1 Hz resolution for the FFT which clearly shows the frequency content, up to 64 Hz. We then present the program with a number of EEGs (currently in the hundreds) and allow it to compute the population statistics of the input samples. In this regard, it is similar to QEEG.
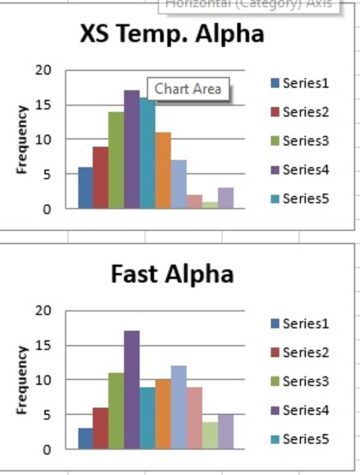
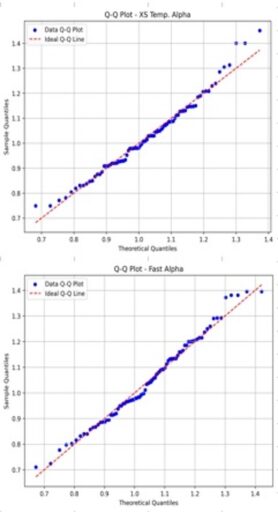
We select EEGs by the fact that they were submitted by a client subscribing to an EEG quality review service, and reported on by a board certified clinical neurophysiologist/QEEG Diplomate, in consultation with 1 or 2 other board certified QEEG diplomates.
The purpose of the doctor’s report is to comment on the quality of the EEG submitted, and make any relevant clinical observations regarding severe abnormalities, or EEG quality problems. Therefore, this is not a “normative” database, but rather one that represents what is seen in a clinical EEG service, and analyzed with regard to what a visual inspection of the EEG might state.
We constructed reference databases from two sets of data, which have samples sizes of 95 and 190 respectively. We observed that the larger database produces slightly wider acceptance bands, but does not affect the resulting z-scores
NOT AI
This system provides automated screening of EEGs, but it is not AI. With AI, a computer learns to recognize patterns based on presented information. This method uses predefined criteria based on years of experience with EEG, and the design of the software has been guided by an experienced scientist who defines the analysis used. Then, by applying the analysis to hundreds of EEG’s, the system learns what is typical and what is not typical, and precisely how deviant any findings are. It is thus useful for tracking changes, or seeing the effects of tasks, or other changes or interventions.
NOT QEEG
Although the BrainPanel Method uses z-score to quantify EEG properties, it is not QEEG. We do not rely on maps or complex graphs, and we do not attempt to compare to a “normal” population. This is an automated EEG quality analysis, pure and simple.
As a form of quality control, and as an assist to a clinical neurophysiologist, this system provides a level of automation that eases the burden when reading EEGs on a routine basis. This tool provides an aid to visual inspection, but does not replace any human tasks required for sound EEG analysis, or preparation for further processing.
In addition to metrics reflecting relative amplitudes and distributions of key metrics, the report analyzes “moments” which reflect the total size of a component amplitude, as well as its distribution within and across the recording session. Extreme moments tend to reflect drowsiness and other changes across a recording, showing changes across time. These are similar but not identical to reliability measures such as split half and test-retest reliability.
This is an example of a machine-generated report in the format of a clinical EEG quality review, produced entirely without human intervention as an automated screening tool for EEG. All EEGs submitted to stseegscreening.com will be given this analysis as part of the routine clinical quality inspection procedure and reports. (Patents applied for)
EXAMPLE MACHINE GENERATED REVIEW SUMMARY
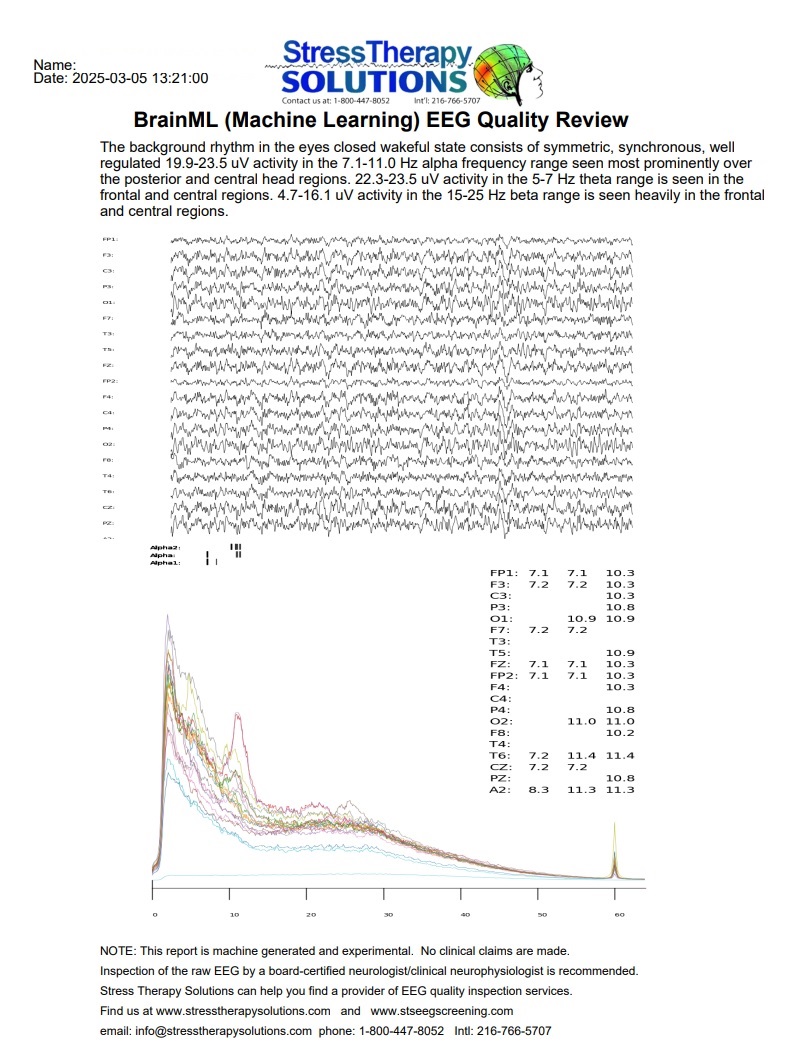
For further information ► https://stresstherapysolutions.com/eeg-screening-review/
Tom Collura
Ph.D., MSMHC, QEEG-D, BCN, NCC, LPCC-S, Founder
“The first rule of any technology . . . is that
automation applied to an efficient operation
will magnify the efficiency.
The second is that
automation applied to an inefficient operation
will magnify the inefficiency.“
— Bill Gates
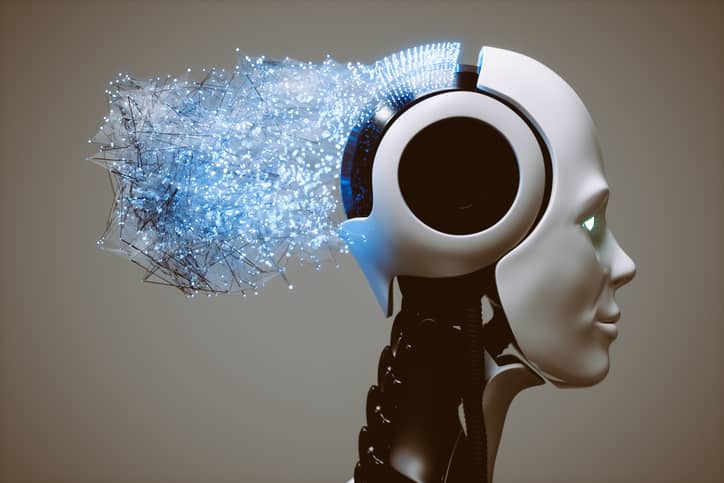